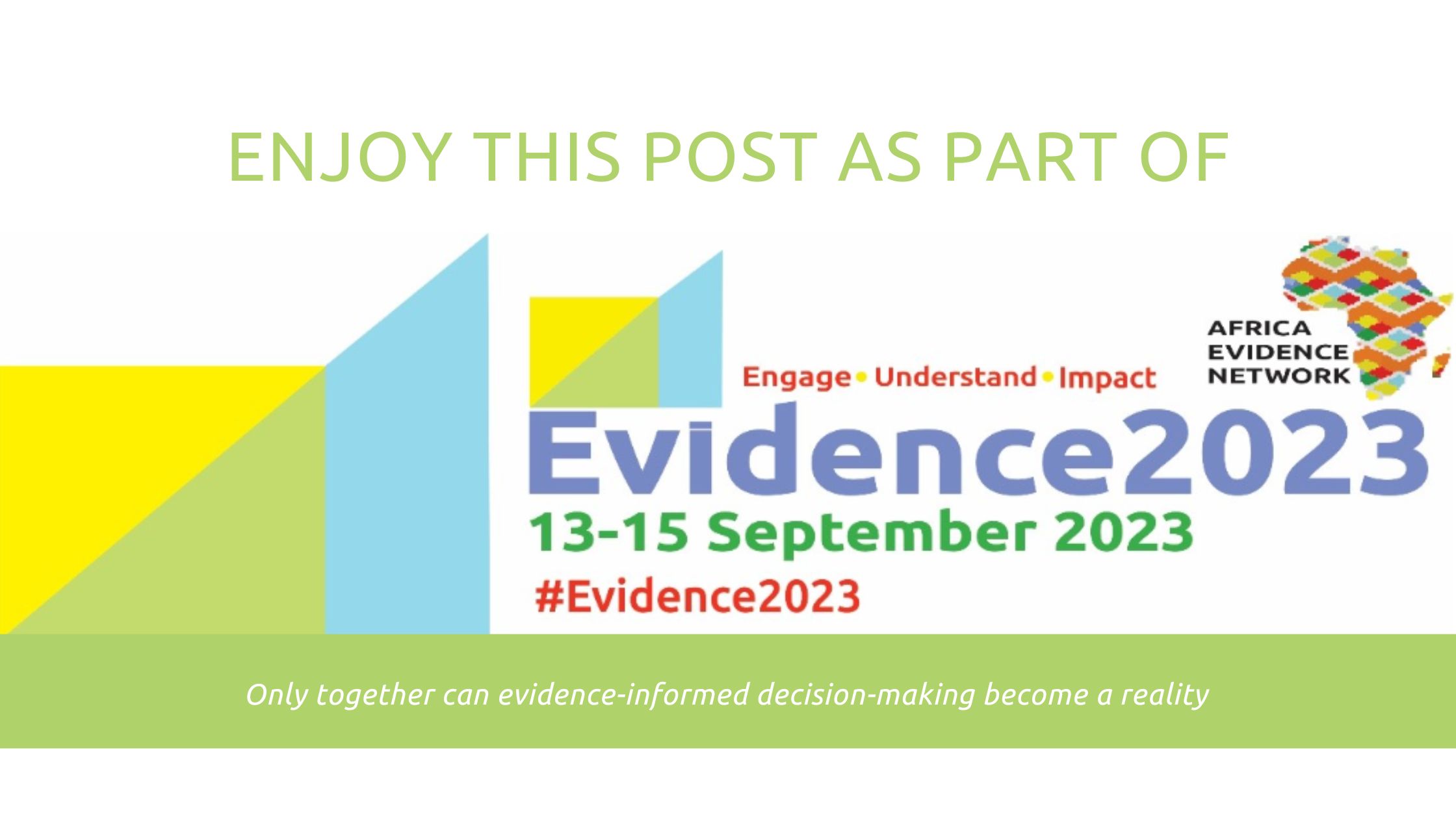
Cancer causes an immense and growing burden, in particular in Africa where limited policies and systems are in place to prevent and manage cases. Effective policy making in cancer requires listening and adapting to the local population’s needs. There is an increasing interest globally in using real world data to inform policy decisions, but this has not yet been explored for policy in the Ghanaian context.
We previously conducted a critical interpretive synthesis on access to cancer treatment in Ghana (Tuck et al., 2022), which revealed that a major challenge is poor patient engagement, as patients often drop out from treatment. It also highlighted a gap in understanding what influenced this, particularly in the five northern regions of Ghana. In our ongoing study, we sought to understand what real world data sources are available (using the northern region of Ghana as a case study) and how these can be use to inform future policy and practice decisions for cancer treatment and care in Ghana.
In 2021 Tamale Teaching Hospital (TTH) established its first specialised oncology treatment centre, which has paved way for cancer treatment in Northern Ghana. Discussions with the centre management highlighted treatment retention poses a challenge as patients frequently drop out from treatment. The reasons for this are not clear but could be due to costs, and also distances. Chemotherapy and surgery are offered at the TTH centre, however patients requiring radiotherapy, or radiotherapy concurrently with chemotherapy, are referred offsite to larger cities in southern Ghana, Accra or Kumasi. In terms of data sources, digital records are routinely collected but have not been operationalised as evidence for decision making so far. In collaboration with local researchers and practitioners at the TTH this co-design study assesses the feasibility of using digital patient records to understand social, economic, and demographic factors influencing cancer treatment engagement in Northern Ghana.
Routinely collected cancer treatment data from November 2020 – 2022 was accessed and extracted from the patient recording system, cleaned, and analysed. After data check and duplicate removals, 493 cases were included in the final dataset.
Through multiple discussions it became clear the treatment pathway was non-linear, dynamic, and of a decentralised nature, making it hard to document and assess with standard measures. We therefore defined and coded an outcome variable that was a composite measure of treatment engagement designed following a treatment pathway mapping exercise. This took into consideration: clinical judgement, digital patient records, and follow up of offsite referrals. The follow ups were made by clinical staff speaking the local language and were met positively by the recipients.
Our analysis of the data revealed a high level of non complete treatment engagement. Less than one third(27%) of patients completely engaged with the treatment as they were advised. Low levels of treatment completion (41%) extended to breast and cervical cancer despite being covered by the national health insurance scheme. For these cancers a large majority of patients' records show that they dropped out due to the financial challenges. This strongly brings to question whether the NHIS coverage is adequate or occurring in practice.
The finding highlights the need for further exploration of why low treatment completion is occurring and what can be done about it.
In further analysis, we wanted to understand how social demographics (age, sex, region, education, occupation) and health related variables (treatment type, cancer, stage, insurance status) impact on treatment outcomes, because our earlier analysis suggested that low social economic groups, those who are uninsured, late stage, from further distance regions, requiring multi-component treatment and not more common cancers (breast or cervical) are less likely to complete treatment. Only treatment modalities (requiring surgery only) and insurance status were significant in the multiple variable model.
We also explored more advanced techniques to look at how social characteristics may act together. For example does the effect of social economic status have a stronger effect in females or a particular region? The approach termed MAIHDA (Multilevel analysis of individual heterogeneity and discriminatory accuracy), can be effective show how different social traits act together. However, it is still a very new technique and few studies have applied hospital data. We found a larger complete data set was required to draw conclusions with this approach.
Due to the decentralised nature of the treatment pathway there was a high degree of missing data (the outcome was missing for 45% of cases). For example, when patients were treated in other settings, the records were not available making it hard to clinically judge.
To better understand the impact this has, we applied an approach called multiple imputation. This used the characteristics we observed about the patients to predict the probability of the possible outcomes for the variables that were missing. Then, we randomly sample using these probabilities multiple times to create multiple imputed datasets. Through considering the variability between variables within each dataset and between each dataset, this approach is able to take into account the uncertainty in using this dataset to make any predictions, making it the most prudent approach. However, this assumes the missing data was not influenced by some unobserved variables for which we are unaware, but can create bias.
The missing data analysis helped to understand the level of uncertainty around the results. Even after accounting for this uncertainty, the level of treatment incompletion was still very high. The imputed dataset predicted only a third (34%) of patients to complete treatment.
This study has led to significant insights in the nature of treatment incompletion and offers many exciting opportunities for multi-sectoral research to inform policy. It involved work between clinical and research staff. Having the clinical input was critical, as in allowed the researchers to fully understand the dataset and how to interpret the findings. However, larger complete data sets would be helpful to gain better understand the effect of social demographic factors that may influence patient engagement. We are now working together on further research to discuss with a wide range of clinical key informants to understand the patient pathway from multiple perspectives and how data from multiple settings can be operationalised for inequity informative decision making.
With many thanks to all staff at Tamale Teaching Hospital, Northern Regional Health Directorate, University of Shefffield and University of Ghana who have supported and input in the project.
Acknowledgements: The author(s) is solely responsible for the content of this article, including all errors or omissions; acknowledgements do not imply endorsement of the content. The author is grateful to Siziwe Ngcwabe and the Africa Evidence Network team for their guidance in the preparation and finalisation of this article as well as their editorial support.
Disclaimer: The views expressed in published blog posts, as well as any errors or omissions, are the sole responsibility of the author/s and do not represent the views of the Africa Evidence Network, its secretariat, advisory or reference groups, or its funders; nor does it imply endorsement by the afore-mentioned parties.